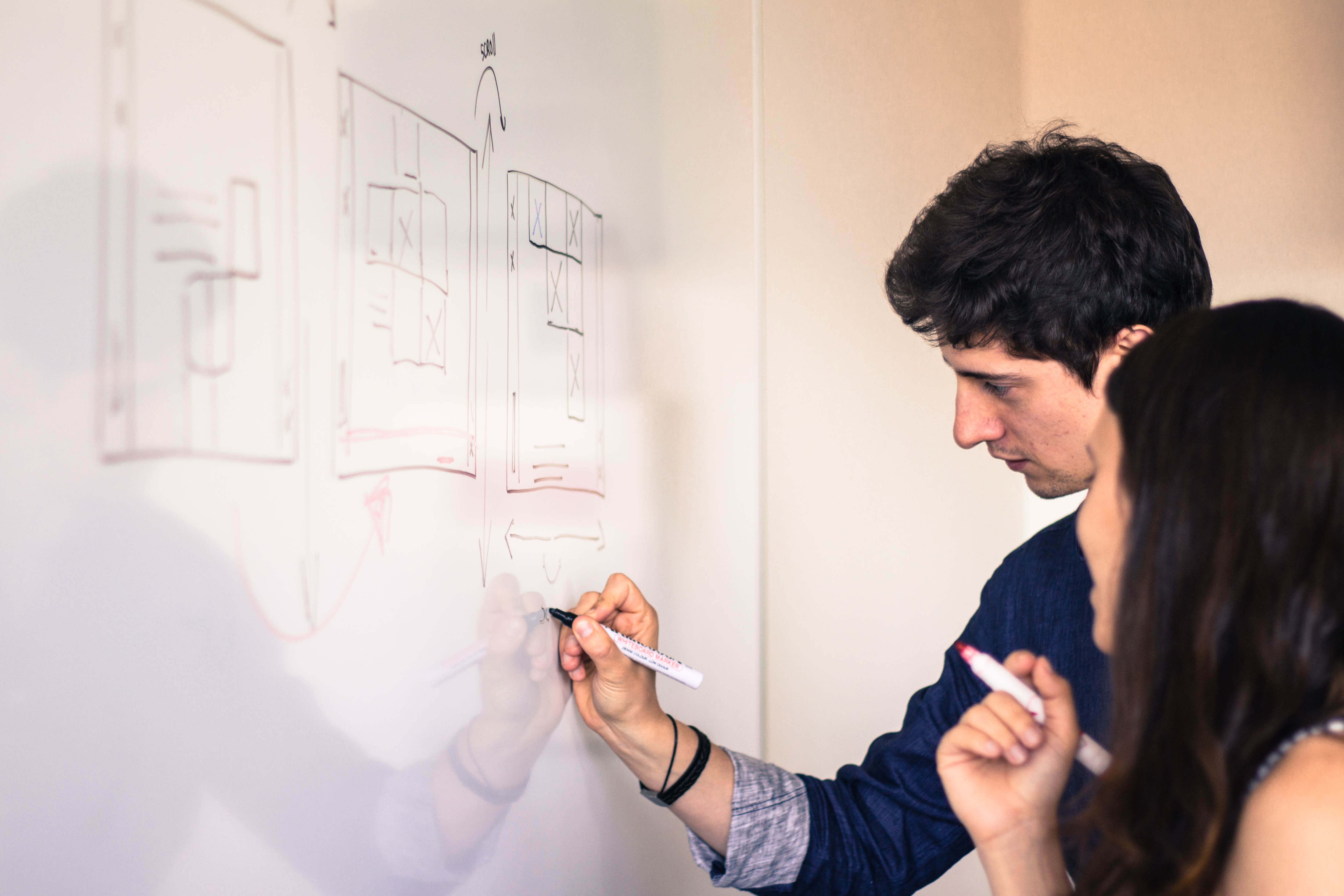
The first step to a successful product strategy is clearly defining what success looks like.
Now that we have defined our strategies, we must understand how to quickly and cheaply validate them.
In this essay, I will not focus on the execution and tactics, which depend on the product and the teams we’re working with, but rather on the principles to assess if our assumptions are correct and we are on the right track.
To achieve that we need to clearly and unambiguously define success, or what determines that our assumptions are correct and our strategy is on track to deliver a valuable and viable product.
At this stage, we already know the company and product’s high-level metrics that our product strategy should positively impact. However, these high-level metrics, such as growth, retention or LTV, often take time to show significant change. Therefore, we need different criteria that are more sensitive to the changes we make to the product, allowing us to gauge success quickly and course correct if needed. Only after this crucial step, we can plan and implement tactics or if you prefer the individual steps to validate our strategies and hopefully contribute to the company or product’s high-level goals.
This is where proxy metrics come into play, as we use them to define the success of our strategies. In statistics, a proxy variable is not directly relevant but it is a substitute for a difficult-to-measure variable due to its correlation. Essentially, proxy metrics are easier to track and impact, and due to their correlation, moving a proxy metric should move your high-level metric. The challenge now lies in defining the right proxy metrics for each strategy. We need to prove their correlation with the high-level strategic metric and, ideally, later on, establish causation. This investment is important because working on the wrong proxy metric can be expensive, time-consuming and have a high opportunity cost.
While correlation does not imply causation, it is the quickest way to identify a relationship between a proxy metric and a high-level metric, so we can quickly start implementing tactics, validating strategies, and later on working on causation.
The process of defining success implicitly starts while we are creating strategies. Strategies are based on data and insights and are hypotheses of the problems we will solve to optimize value and viability. Technically in this process, we are theorizing about a relationship between strategies and the value created. Defining success is defining how we prove that relationship.
Going back to the project we’ve been using as an example, one of our strategies was “easy and quick ordering” which should contribute to our high-level strategic growth metric - new customer growth YoY. So, how can we prove this? As you all know, moving this high-level growth metric is difficult, so we had to find ourselves a good proxy metric to measure impact and assess if we were moving in the right direction.
While we were crafting this particular strategy we collected insights from market data, competitor and technological analysis, customer data and learnings from customer research, interviews, and surveys. During this process, we researched our unsuccessful leads within the targeted segment, which raised several questions. Why some of our most promising leads did not complete their orders? Are they unhappy with our interface? Do we lack the products they are looking for? Are they confused with the flow?
As we dug and started interviewing these unsuccessful leads we understood that these leads rarely pursued orders that took too much time to be confirmed. This valuable insight pointed us in the right direction and allowed us to look into our data and understand that there was a negative correlation between the time to confirm an order and the success of said order. Digging even deeper into this issue we later understood that this was also true for sales calls, if the order confirmation took too long to confirm, the probability of that lead to churn would be higher. We also discovered a direct correlation between months of higher growth and the average time taken to confirm an order.
So the proxy metric naturally came into play to guide this strategy: “percentage of new customers that had their order confirmed within one minute”.
This particular metric had some important characteristics that make it a good proxy metric for us, we had historical data on it, we could prove correlation with the high-level growth metric through data available, was within our control, was targeted to new customers and we could track its progress daily. After having success defined, and our proxy metric settled, we started planning and implementing tactics that allowed us to validate our assumptions and the results quickly appeared.
Within a month our proxy metric increased by 20%, and we started seeing strong evidence of its positive impact on the high-level growth metric, particularly in the cohort where the tactics were in effect. To be completely honest, we were so convinced that this was the right approach that we implemented this change quickly for all the customers without further causation analysis, as we strongly believed that the tactics implemented would improve the customer experience and in this case customer growth.
In this particular example, we didn’t do further causation analysis but in many cases, proving causation is necessary. As Gibson Biddle mentions, “Long-term, you hope to prove causation via a large-scale A/B test,” or through other tailored frameworks.
Another note to take into account is customer segments and cohorts. I haven’t delved into specific details, but different customer segments may require different tactics and strategies. For instance, in the example above, easy and quick ordering was not a problem for some segments, but instead, the support quality - another strategy in our pipeline. This demonstrates the importance of thorough research around your customer segments in your product strategy. If we had analyzed all segments together, we wouldn’t have identified the negative correlation between order confirmation time and order success that guided this strategy
Don’t forget that sometimes your assumptions and strategies are wrong, and you need to course correct. That’s why it is so important to have clear success criteria, so you don’t spend time and money on things that will not impact your business and product. I assure you, many of your strategies and hypotheses will be off the mark (as many of mine were!), but this process allows you to fail fast and learn quickly. Rule of thumb if it doesn’t work, switch quickly, don’t lose time. Never forget that your product strategy is a learning experience, you’re supposed to learn.
In conclusion, the most crucial aspect of this process is your mental model. Your product strategies are mostly assumptions that you need to validate. So before you think about features, tactics or anything to implement, you need to think about the problems you want to solve (strategies), and how these will contribute to delivering a valuable and viable product. A common pitfall of this process is to create proxy metrics and strategies based on a feature roadmap, which defeats the whole process. The tactics you implement are secondary, they are the means to achieve a goal.
In the next essay, we will talk about how to communicate your progress to the relevant stakeholders.
Updated Index of all articles released so far:
The Non-Technical Founders survival guide
How to spot, avoid & recover from 7 start-up scuppering traps.
The Non-Technical Founders survival guide: How to spot, avoid & recover from 7 start-up scuppering traps.