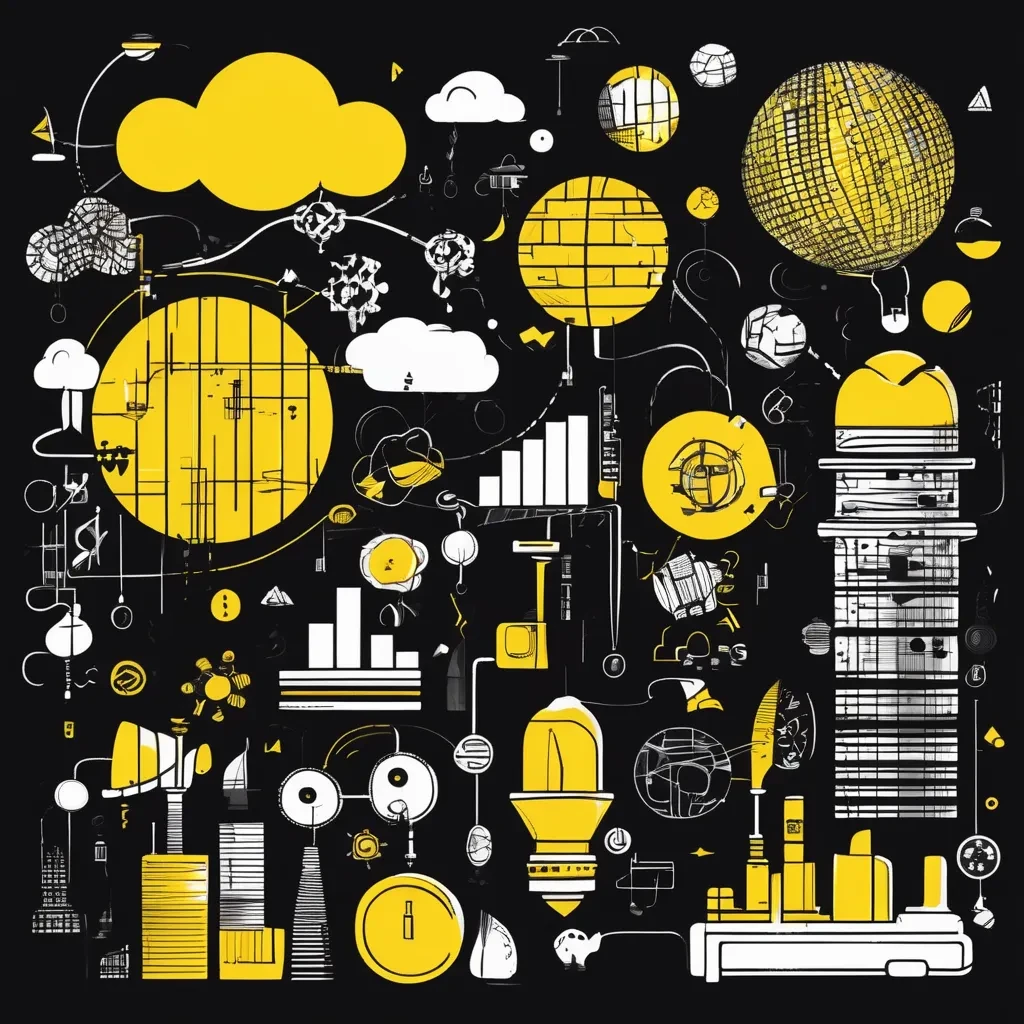
Analysing legal documents for carbon credit assessment presents significant challenges for multiple stakeholders. This article explores how we transformed this complex process through Generative AI implementation, following a structured approach to deliver meaningful results.
Research & discovery
We started with a comprehensive analysis of a specific step of the carbon credits certification process, where we identified key pain points and opportunities for improvement. Through stakeholder interviews and process mapping, we discovered that one of the most time-consuming and resource-intensive tasks was analysing and interpreting legal documents related to carbon credits.
The research phase revealed that our client classified the task of assessing carbon credit feasibility across different markets and activities the most tedious and time consuming. This understanding helped us identify where Generative AI could make the most significant impact in streamlining operations.
Use case development
Based on our initial findings, we developed a specific use case focused on reducing the time required to analyse legal prerequisites for carbon credits in designated countries and activities. We experimented with different AI models, particularly Claude 3 Sonnet and Haiku, to evaluate their document analysis capabilities and determine the most effective solution.
The ROI analysis showed promising results:
- Average cost of 0.12€ per legal document analysis
- Significant reduction in processing time
- Enhanced accuracy in requirement extraction
Prototyping
We developed a proof-of-concept solution with key functionalities:
- Automated analysis of legal documents related to carbon credits
- Extraction and compilation of requirements
- Cross-referencing capabilities
- Interactive follow-up inquiry handling
- Document upload and analysis features
The prototype was tested using 10 documents relevant to carbon credits and forestry, with Claude 3 Sonnet demonstrating strong capabilities in information extraction and relevancy determination.
Impact and results
The implementation has shown promising results in terms of:
- Cost efficiency: Average processing cost of 0.12€ per document
- Time savings: Significant reduction in document analysis time
- Accuracy: High-quality extraction of requirements
- Scalability: Ability to handle multiple countries and activities
We are now in a stage of feedback collection to ensure the next steps of development are aligned with the business needs.
This AI-driven transformation represents a significant step forward in legal document analysis for carbon credit assessment, demonstrating how targeted Generative AI implementation can address specific business challenges while delivering measurable results.
Applying this framework to other processes
The success of this AI implementation in legal document analysis provides a blueprint that can be readily adapted to various business processes. Whether it’s contract review, financial report analysis, technical documentation, or customer service operations, the same structured approach of thorough research, focused use case development, rapid prototyping, and systematic implementation can significantly improve efficiency and accuracy.
The key to a good adaptation lies in identifying processes with similar characteristics: high volume of document processing, repetitive analysis tasks, and clear success metrics. Organisations can achieve comparable benefits across different departments and functions by following the core principles of careful process assessment, appropriate technology selection, and measured implementation strategy. This framework’s flexibility, emphasising practical implementation and continuous improvement, makes it a valuable tool for any organisation looking to leverage Generative AI for process optimisation.
The Non-Technical Founders survival guide
How to spot, avoid & recover from 7 start-up scuppering traps.
The Non-Technical Founders survival guide: How to spot, avoid & recover from 7 start-up scuppering traps.